

Hope this information helps in understanding EMR and Redshift use cases better. Powering Amazon Redshift Analytics with Apache Spark and Amazon Machine Learning Build a Healthcare Data Warehouse Using Amazon EMR, Amazon Redshift, AWS Lambda, and OMOP How I built a data warehouse using Amazon Redshift and AWS services in record time I have tried to check some of AWS blogs which shows how EMR and RDS can be used together in specific use cases. I am listing other resources which can help to understand RDS and EMR use cases better.ĪWS redshift related case studies > Look for case study section :ĪWS EMR related case studies > Look for case study section : Q: What is the data processing engine behind Amazon EMR? Q: What can I do with Amazon EMR that I could not do before? Q: Can I use Redshift Spectrum to query data that I process using Amazon EMR? Q: Can Redshift Spectrum replace Amazon EMR? Please check below Redshift specific faq: Watch this meetup video to understand in depth Big Data Architecture conciderations in AWS.
#Aws emr vs s3 copy log files to redshift code#
Notebook built in – mix your code with SQL via Zeppelin.Orchestration built in such as Oozie, although Airflow is more common.Complex partitions + dynamic partitioning + insert overwrite.this is cloud architecture best practice. When you want to decouple compute and storage ( external table + task node + auto scaling).When you data scales until a few hundred TB’s.When cost is important: spot instances.When compute elasticity is important ( auto scaling on tasks).When you need a transient cluster, for night or hourly automation.When you want analize massive amount of data ( spectrum).When you data type are simple, i.e not Arrays, or Structs.When you need the data relatively hot for analytics such as BI.AWS Region: N Virginia Number of AWS Data Pipelines: 2 Source RDS Instance name: srv Source database name( default): mydb Source table name: emp emp table structure: empname varchar(20), address varchar(20) Number of records in emp: 2 Destination RDS Instance name: dst Destination database name( not default and exists): bank Destination table name (doesn't exist): emp S3 bucket name: dpl11 S3 File format. csv file to emp table in the bank database of dst RDS instance. The second Data pipe line c should push contents of this. Also we are not using default VPC which again require additional configuration which also is documented here.įirst Data pipeline should be able to pull data from emp table in the mydb database of srv instance and store in an S3 bucket ( dpl11 ) in the form of. The dst RDS instance has one database with name bank (which is not default database created at the time of RDS instance- for this reason we do some additional configuration in the experiment - see configuration below). For the purpose of experiment two records are inserted into this source table emp. The srv RDS instance has a database mydb and table named emp within it, which has only two fields. We will create two RDS MySQL instances one with name srv and other dst. csv file back to to another RDS MySQL instance. Here we experiment how AWS Datapipeline can be used for copying RDS MySQL table to S3 in. During the process you can also see creation of intermediate resource like launching of EMR cluster or EC2 instances triggered by the AWS Data pipeline components Task Runner and Pipeline Definitions. AWS Data Pipeline one of the solutions which can be used automate the movement and transformation of data.
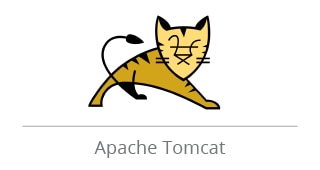
It could be the user require to backup the data to a durable and cost effective medium like S3, or it could be the user need to copy the text based data from S3 to databases like RDS, Redshift or dynamodb.
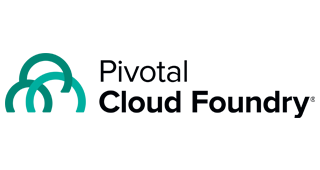
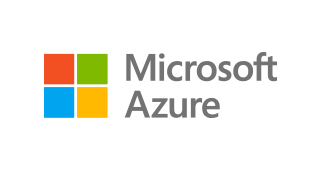
Sometimes, it is necessary to copy the data between different AWS compute and storage services, or on-premises data sources. AWS Data Pipeline copy from RDS MySQL to S3 and Back
